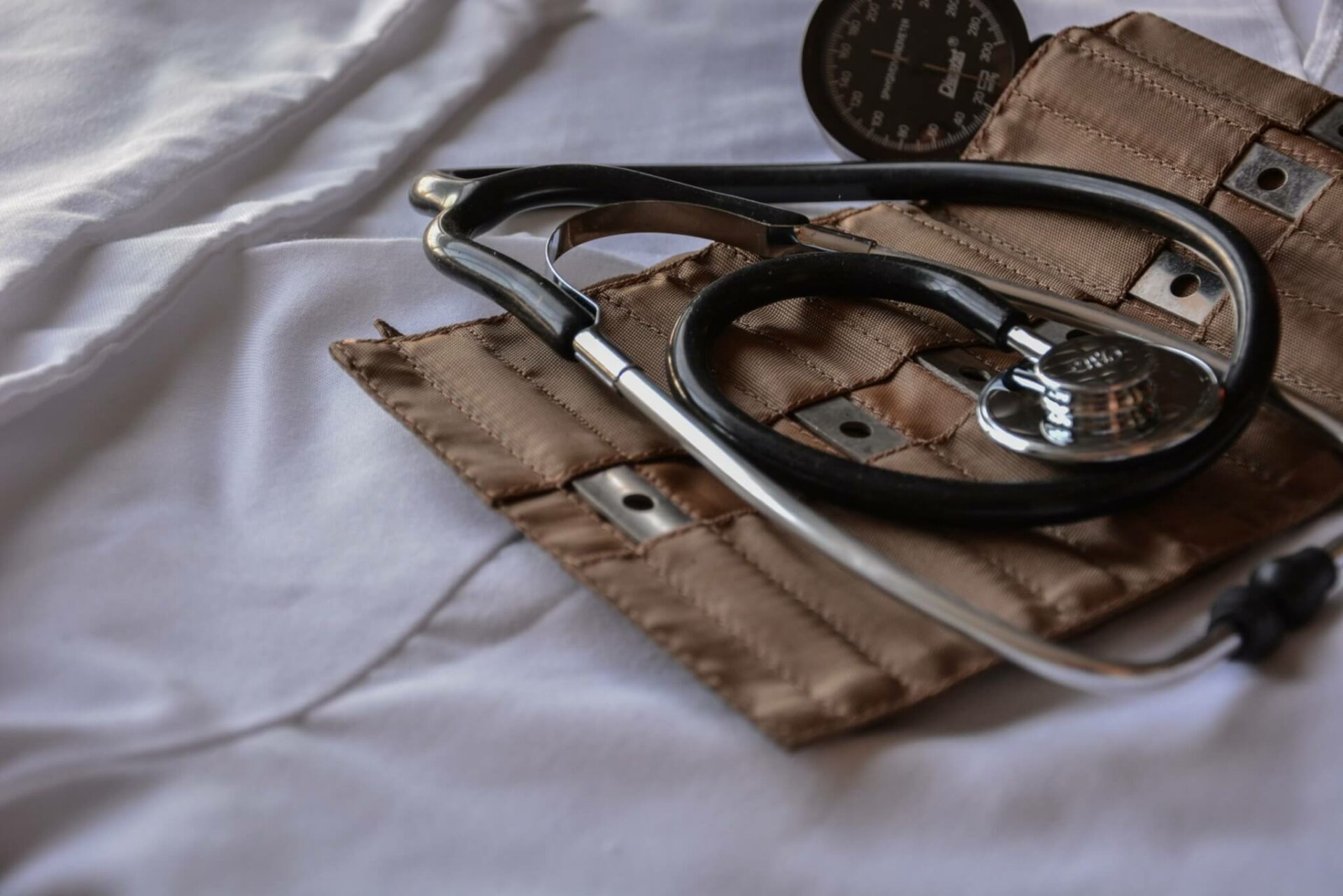
In Part 1 of our series we introduced the rare disease (RD) ecosystem, which is defined by a small patient population with often heartbreakingly high unmet need. Here, in Part 2 of our series, we will discuss in more detail the overarching design requirements and key considerations for RD clinical trials.
The entrance of a new therapeutic via a clinical trial is a big deal in RD, with high stakes for patients and caregivers as well as RD companies taking a risk on entering an unproven and often poorly-developed market. In addition, unlike other disease areas in which companies often develop pipelines in the same therapeutic area and can cultivate relationships with key stakeholders over time, an RD company introducing a new therapeutic via a clinical trial is also introducing itself to the community for the first time. Therefore, first impressions are especially critical for clinical, regulatory, and eventually commercial success.
As to be expected within the RD ecosystem, clinical trial design faces certain selective pressures, namely small patient population size, high unmet need, limited or no standard of care / comparators, and the uniqueness of the diseases themselves which means validated endpoints may not yet exist. Together, these factors make the traditional design of large, randomized, controlled clinical trials with standard endpoints impractical if not impossible for rare diseases. Instead RD clinical trials tend to be “small and smart”, with minimal cohort sizes, streamlined designs that favor participants to receive the therapeutic, and novel endpoints that appropriately reflect a therapeutic’s efficacy and desired outcomes.
In addition to adapting to these selective pressures, an RD clinical trial must convince stakeholders to engage at one or both of two levels: first, to participate in the trial and second, to be convinced by the data generated out of the trial. RD companies should therefore proactively engage various members of the RD ecosystem during the design stage of clinical trials. The following stakeholders are particularly important:
- Patient Advocacy Groups, Patients, and Caregivers who can speak to unmet need and meaningful outcomes
- Centers of Excellence (COE) / Key Opinion Leaders (KOLs) who will be doing the work of running the trials and communicating outcomes, who can speak to both practicality and robustness of proposed trial designs
- Regulators who will make the final decision on whether trial outcome data is sufficient for regulatory approval
- Payers who will make value-based coverage decisions (at a potentially high list price)
RD Clinical Trial Design: Unique Considerations, Challenges, and Opportunities
While RD clinical trial design has the same goals as any trial would (i.e., defining dosing, safety, and efficacy), RD companies face specific challenges and opportunities as they work through key design considerations. RD trials are typically very small, with limited datasets that must play an outsized role in convincing key stakeholders. So, RD trial design considerations are tightly interrelated, typically requiring an iterative design process that involves key stakeholders early and often.
Both anticipated and unanticipated questions will arise during the design process. RD companies should take advantage of key stakeholders’ expertise to work through them in order to craft the most successful designs.
1. Patient Population Definition
To plan a clinical trial, one must first define the patient population. This is not always straightforward in rare diseases, as many lack consensus on consistent staging or grouping (or sometimes even on diagnostic criteria); thus the RD company may find itself doing upfront work to articulate how to identify the patient population.
Typically, this will include some combination of a known diagnosis, a mutational profile, and / or some measure of disease burden (e.g., functional status). Importantly, genetically-driven rare diseases may not be fully penetrant (meaning that people with the same mutations may have highly variable disease burdens). So, while it may seem straightforward to identify eligible trial participants based on mutational profiling, in practice it may not be sufficient.
RD companies in a more competitive space must also consider how their defined patient populations intersect with competitors. For example, Biogen’s SPINRAZA was approved in Spinal Muscular Atrophy (SMA) based on a trial for “symptomatic patients” with no mention of genetic profiling, whereas Novartis’ ZOLGENSMA was approved in SMA based on a trial for patients “with bi-allelic deletions of SMN1, 2 copies of SMN2, and no c.859G>C (exon 2) mutation of SMN2” – a very specific mutational profile but no mention of symptoms. Both ultimately obtained a similar label, but the greater specificity of Novartis’ defined population may have had trial enrollment implications.
Finally, for those rare diseases which are present at birth, RD companies must also consider which minimal age or weight restrictions are appropriate to best determine dosing, safety, and efficacy.
Key Questions and Opportunities to Engage Key Stakeholders
COE / KOL / Academia:
-
- Is the “appropriate” patient population already well-defined and easy to identify in practice? (If not, let’s work together to define it)
- Based on the therapy’s expected mechanism of action, are there additional inclusion or exclusion criteria that should be considered?
Regulator / Payer:
-
- Is the proposed patient population definition sufficient for label and coverage decisions? If not, how might we expand or adjust the population?
- Does it allow for potentially broader use and coverage? If not, what evidence plan could support this?
2. Endpoint Selection
Ultimately, the goal of the clinical trial is to determine efficacy of the therapy as measured by pre-determined endpoints that measure disease status and progression. In RD, efficacy endpoints can range from more objective to more subjective, for example biomarkers, functional scales, and patient-reported outcomes or caregiver assessments, respectively. When considering endpoints for a clinical trial, the RD company must weigh which type of endpoint is best positioned to confirm clinical benefit, also taking into account the amount of work required to validate novel endpoints they are proposing. Because of the uniqueness of each disease, RD companies have both an opportunity and a challenge to define and validate endpoints which are:
- Measurable (i.e., as objective as possible) for KOLs / COE running the trials
- Meaningful to patients / caregivers / Patient Advocacy Groups
- Sufficiently validated for Regulatory and Payer decision-makers
This can be a delicate balance: three recently approved Duchenne’s Muscular Dystrophy (DMD) therapies were approved based on biomarkers, i.e., demonstrated increases in dystrophin levels, which was acceptable to regulators but led to some controversy as patients/caregivers and Patient Advocacy groups found this easily quantifiable but indirect measure less compelling than direct functional measures would have been.
Conversely, while functional outcomes and particularly Patient-Reported Outcomes (PROs) are appealing because they are the most direct measure of meaningful outcomes to patients, they also tend to be more subjective and harder to measure. An RD company should keep in mind that a reliance on PROs as a primary endpoint will likely necessitate a bigger cohort size and more oversight in order to collect usable data.
Another consideration is whether and how to include multiple endpoints, given the complexities of RD biology. For example, in Huntington’s Disease, the level of mutant huntingtin is a well-defined biomarker, but the clinical manifestations of disease can be quite variable, making functional endpoints more challenging but also important to measure so that a more comprehensive picture of efficacy emerges. Currently, the FDA and other regulatory bodies prefer a single primary endpoint to streamline their assessment of efficacy, but it is relatively common to include a mix of biomarker-based and functional status-based endpoints across primary and secondary endpoints.
RD companies should also consider the acute impact of COVID-19 and the more general trend towards telehealth and remote monitoring, as well as travel burdens on a broadly-distributed patient population, as they define endpoints with an eye on decreasing the need for in-person appointments. For example, consider endpoints that can leverage wearable tech (e.g., EEG measures), video recordings to assess functional status that are done at home and submitted electronically to the trial sites, etc.
Key Questions and Opportunities to Engage Key Stakeholders
COE / KOL / Academia / Regulator / Payer:
-
- Is this measure acceptable to you as proof of therapeutic effectiveness? What validation (if any) is required?
- If other endpoints have already been introduced by competitors, what concerns (if any) do you have about those? How preferable is it that we include the same to allow data comparisons?
- Are anticipated safety concerns adequately addressed by planned monitoring?
Patient / Caregiver / Patient Advocacy Group / HCP:
-
- Is this measure meaningful to you as proof of therapeutic effectiveness? What validation (if any) is required to show it correlates with a true benefit?
- Do you have concerns about the feasibility of participating in data collection (e.g., requirement to visit trial sites, invasive monitoring)?
- If other endpoints have already been introduced by competitors, what concerns (if any) do you have about those? How preferable is it that we include the same to allow data comparisons?
- Are anticipated safety concerns adequately addressed by planned monitoring?
3. Comparator Selection
Given the high unmet need of rare diseases and frequent lack of a standard of care, RD companies have an ethical responsibility to maximize the opportunity for clinical trial participants to receive the therapeutic. That said, they also must be able to demonstrate a compelling and statistically significant analysis of safety and efficacy, which requires some sort of comparator. There are four common comparator designs used by RD companies to achieve these parallel goals:
- Single arm design: In this design, all participants receive the therapeutic, and the data is compared to either the participant’s starting condition, or to a separate synthetic comparator (e.g., historical controls). It may be harder to detect (or convince stakeholders of) a compelling efficacy signal, depending on how the comparator is defined. This design is suitable for initial signal-seeking trials and in some cases may be sufficient for accelerated approvals (which must subsequently be confirmed with more robust datasets).
- Dosing cohorts: In this design, there are two or more dose-based cohorts so all participants receive the therapeutic, but the internal comparison between pre- and post-treated and between lower and higher doses can support dose-dependent safety and efficacy analysis. This is suitable for signal-seeking trials but is typically not sufficient for registrational trials.
- Cross-over design: In this design, some participants start out as part of a control arm, but at a predetermined point (either progression-based or timepoint-based) they switch over to receiving the therapeutic, in effect becoming their own comparators. Sometimes, the cross-over is not part of the trial, but instead all participants are offered the opportunity to continue after the trial with a separate open-label extension trial in order to receive the therapy.
- Weighted assignment: in this design, participants are still assigned to one of the classic two arms of therapeutic vs. control, but the ratio is set so that most participants will receive therapeutic while still enabling a statistically-solid analysis, for example 2:1 or 3:1. This trial design can also be combined with a cross-over to enable all participants to eventually receive the therapy.
These comparator design options may not always be sufficiently compelling to regulators on their own but can be combined into an approvable regulatory package. For example, Novartis’ ZOLGENSMA was approved in SMA based on a data package including a completed dosing cohort trial and an ongoing single arm vs “natural history of the disease” control.
Key Questions and Opportunities to Engage Key Stakeholders
Patients / Caregivers / Patient Advocacy Groups:
-
- Would you join (or recommend) this trial, based on the possibility of being assigned to the comparator cohort?
COE / KOL / Academia / Regulators / Payers:
-
- Are these comparators sufficient to demonstrate compelling safety and efficacy?
- Does this design strengthen our data as compared to competitors who used different comparators (where relevant)?
4. Cohort Size Definition
While some rare diseases are rarer than others, by definition none of them will have a large, easily accessible patient population. RD companies must thoughtfully design their trials to maximize signal detection (both efficacy and safety) with as small a population as possible. Cohort size definition is heavily dependent on an assessment of what n-value will enable signal detection given the anticipated product profile, defined patient population, endpoints, and comparators. However, if the target cohort size based on these parameters is too large to be feasible, the trial design must be adjusted so that the enrollment target is realistic.
Key Questions and Opportunities to Engage Key Stakeholders
Regulator / Payer:
-
- Is data coming out of this trial size / n-value sufficient to enable regulatory approval and coverage decisions?
- How might we adjust the other design elements and still produce a compelling dataset?
COE / KOL / Academia / HCPs:
-
- How realistic are our enrollment targets?
- Is data coming out of this trial size / n-value convincing for you to change clinical practice? What if we don’t quite reach statistical significance?
- How might we adjust the other design elements and still produce a compelling dataset?
Conclusions and What’s Next
RD companies are by definition operating in a small ecosystem, and while there are certainly associated challenges related to disease complexity and rarity of patients, there is also significant opportunity for RD companies to work collaboratively with key stakeholders to rapidly and significantly advance the standard of care. In many ways this begins with the clinical trial design, as the key design considerations are solidified and pressure-tested within the rest of the ecosystem.
In this article, we focused on the design of (pre)registrational clinical trials, highlighting the similarities and unique differences RD companies face in designing clinical trials. However, as they reach the point of entering the clinic, RD companies should also be planning for registrational success and building out their post-registrational evidence generation plans that will bolster ongoing success for their products. We will discuss this in-depth in the Part 3 of our series. In Part 4, we will discuss the implementation of clinical trials (both pre- and post-registrational).