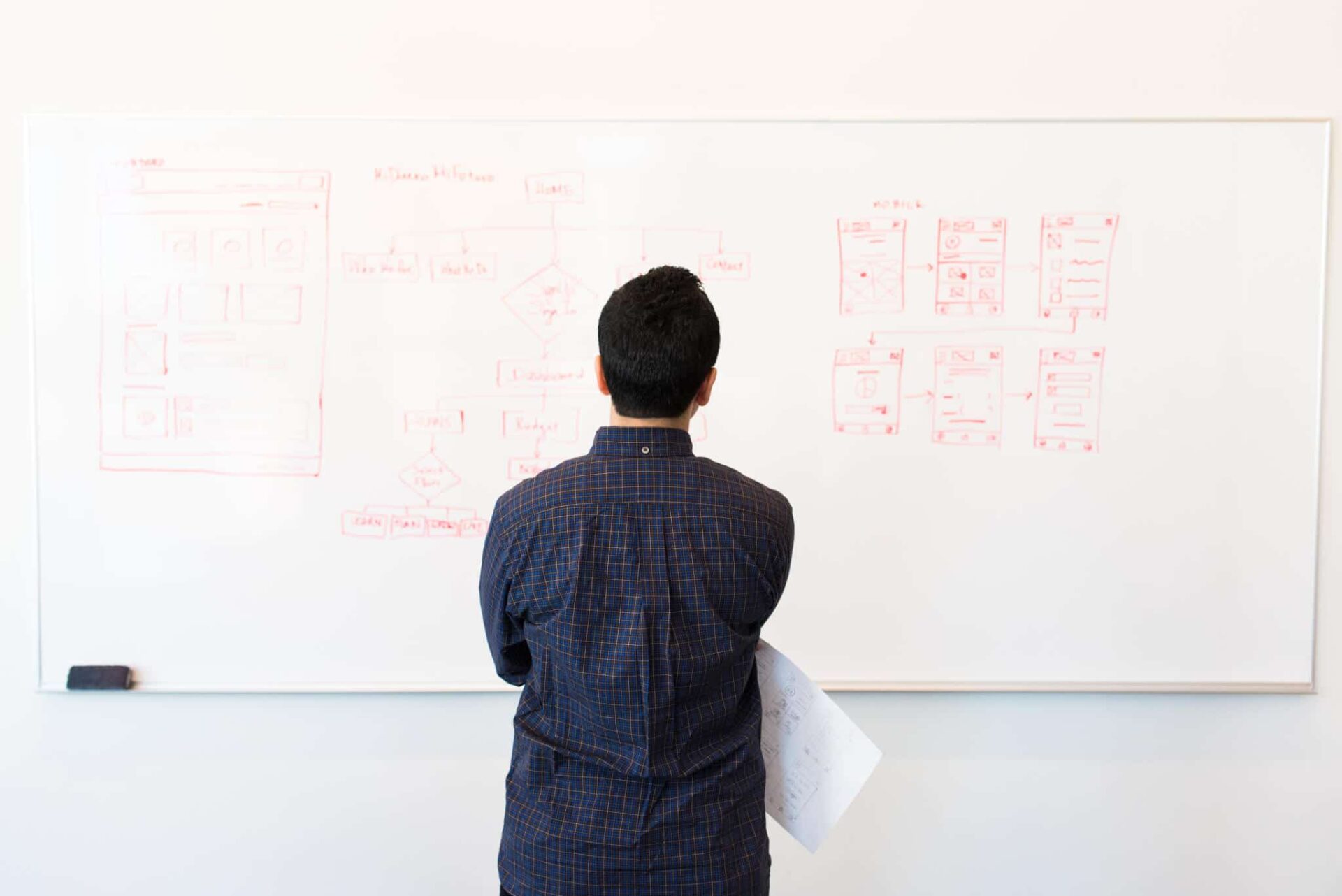
Future Trends
In Parts I and II of this series, we reviewed the dramatic diagnostic and pharmaceutical advances over the past 5 years that have rapidly enhanced the standard of care, leading to significant and essentially curative outcomes for some patients. In this article, we discuss the near-term future – the possibilities as well as the organizational requirements to achieve them. Within the next 5 years, we see the potential to dramatically broaden and extend cancer treatment responses via several key avenues:
Early Disease Detection
Diagnostic-driven disease detection and characterization will enable more cures as oncologists identify and curatively treat cancers at earlier stages (before metastatic spread). This will typically involve classical surgical resection as well as maintenance drug regimens to prevent a recurrence where indicated.
For those tumors that are already advanced or metastatic, diagnostic-driven detection of progression will enable the earliest possible change in treatment and highlight specific mechanisms of resistance. This will enable oncologists to apply the most effective treatments before more resistant tumor cell sub-populations take hold.
More Strategic Treatment Options
Currently, treatments tend to be “one shot and then hope for the best / wait for progression”. By the time progression is detected, typically it has taken hold and become resistant to further treatment. Often, the treatment intent then shifts from curative to palliative.
With more sensitive diagnostics and additional pharmaceutical options, we can apply more advanced regimens that better target the tumor biology through combinations. This includes regimens designed to address resistance mechanisms before they take hold. This will likely include not just concurrent combinations, but sequential regimens designed to leverage sensitization or to suppress resistance mechanisms.
Individualized Regimen Selection
As diagnostics improve and more treatment options become available, we anticipate a dramatic increase in individualized (“personalized”) regimens. Decision criteria will not be limited to just better targeting of the specific tumor biology, but ensuring that the regimen is safe and tolerable for a specific patient, given other considerations like their overall health, age, comorbidities, etc.
Improved Treatment Access
Identifying better treatment options will not be sufficient to broadly improve outcomes. For example, in the current US healthcare system, many patients are unable to easily access or afford treatments not yet part of the standard of care (including clinical trials). We anticipate that regimen selection will accelerate faster than the standard of care, so new mechanisms will be required to support actual access to these treatments. This may include:
Organizational Requirements to Succeed
Market success in this future will require more than just developing effective drugs and easy-to-use diagnostics. As in any market, differentiating from the competition will be critical. Being first in a market or defining unique market niches (patentable designs, specific patient populations, etc.) will become increasingly important.
Success in this highly complex, technically evolving market will require specific organizational capabilities, both now and in the future:
Data Complexity Management
As pharma and diagnostics products are developed and ultimately enter the market—and regimens become more personalized—the amount of data that must be collected, managed, and interpreted will exponentially increase. Successful pharma and diagnostic companies will need to manage data across several dimensions to answer several key, interrelated questions:
Typically, this will be done in a learning loop style where researchers select a patient population based on either pre-existing data about population + regimen pairings or on a new hypothesis. Where relevant, researchers use various diagnostics / biomarkers to select the population, then apply the regimen and track the outcomes.
Each outcome will answer one specific question about a population + regimen, as well as the predictive ability of the diagnostic. However, successful product development and portfolio management will require triangulation from numerous data sets like this in order to identify the most promising approaches. To broaden the success of its portfolio across products and indications, a successful organization must be able to rapidly integrate data sets from both internal data sources (pre-clinical, clinical trials) and external sources once products reach market (phase IV trials, real-world data).
In addition to population and regimen selection, another source of data complexity is biological. There are numerous possible predictive molecular alterations that may be considered for each population and / or regimen. Broad genomic panels and other broad tests will offer an enormous range of possible stratifications, and a wrong selection will lead to a drug’s failure. Similarly, selecting alterations for diagnostic test design must also be done correctly, or the test will not prove clinically predictive. A successful organization will have strong scientific and computational resources to use this data for successful diagnostic and drug design.
Optimized Decision-Making
Of course, successfully collecting and managing complex data does not help unless the organization can effectively leverage it for decision-making. Smaller companies with only one or a few products can more easily align their decision-making to market potential. For example:
For companies with broader portfolios, decision-making becomes much more complex. Organizational resources must be allocated across products and / or indications (depending on the organizational structure). In this situation, it is essential to take a cross-portfolio view of pipeline valuation and pre-determine informed decision points (go / no go, accelerate, initiate development plans in other indications).
To successfully do this, decision-making must at the product level as well as the portfolio level. Resource allocation should incorporate the latest data to adjust the probability of success and the market value for each program. At the same time, organizations must be wary of false precision based on whatever valuation models they use.
Smart Trial Design
Currently, clinical trials are frequently inefficient and costly, from both an organizational as well as a patient-centric perspective. The traditional randomized control design requires that a percentage (typically 1/3 to 1/2) of the trial participants only receive the standard of care treatment.
In intractable cancers, patients must therefore risk being assigned an ineffective treatment, and companies must cover the expense of trial management for this large set of patients with a known detrimental outcome. On the other hand, the early stage dosing trials also pose risks of safety (too high a dose resulting in toxicity) and lack of efficacy (too low a dose that provides no therapeutic benefit).
Diagnostics require a Pre Market Approval process to demonstrate clinical utility before they can receive FDA approval, but tests are frequently sold before then based on their CLIA approval (which confirms that they accurately measure what they say they do, but does not speak to their impact on clinical decision-making and outcomes).
However, there is a high unmet need in many cancer indications and a “need for speed” when it comes to getting more effective diagnostics and drugs to patients. So, development programs are increasingly given the flexibility to design trials outside the traditional model, as long as they can satisfactorily demonstrate safety and efficacy (for drugs), and analytical and clinical validation (for diagnostics). We expect this trend to continue and become the new standard as it delivers both time and cost savings. For example, several recent drugs have received a preliminary approval from early phase studies – most notably Keytruda in 2017 based on Phase 1b trial results.
The most effective clinical trial designs now and in the future will deliver quality results with time and / or cost savings, typically by achieving statistically significant results from a smaller trial population. Several innovative tactics are already in use and showing good success.
Streamlined Recruitment
Both diagnostic and pharmaceutical trials can improve their matching and enrollment process to deliver significant time savings. Currently, potential participants must seek trials either through their treating physician (who is likely unaware of many trials outside their home institution) or directly via the clinicaltrials.gov website, which is not lay person-friendly and cannot be filtered by detailed eligibility criteria. While many larger companies offer their own trial matching services, a centralized resource that covers a comprehensive list of available trials plus a high-level eligibility filter will help drive more patients more quickly to the appropriate trials.
Novel Endpoints
For solid tumors, Progression Free Survival (PFS) or Overall Response Rate (ORR) endpoints use RECIST criteria, which is based on tumor size assessed by MRI or CT scans. However, this approach is challenged by the complexity of metastatic or multifocal disease (which tumor should we measure?) as well as by the patterns of response to certain drugs. Specifically, cancer immunotherapies sometimes show an atypical pattern of pseudo-progression followed by response (because the immune activation around the tumor appears as tumor growth on the scans).
So, the search for other acceptable measures of efficacy is underway. This may include more systemic measures of tumor response or growth such as circulating tumor cell (CTC) levels, or biopsy-based measures of early response such as immune cell infiltration into the tumor.
Ultimately, the key outcome will remain Overall Survival (OS), but these novel endpoints will be beneficial to help organizations make internal decisions about acceleration and prioritization. In the marketplace, endpoints associated with quality of life could also help differentiate from competition.
Efficient Comparator Arms
Instead of the traditional standard of care arm run in parallel with the experimental regimen, companies are exploring multiple types of alternative controls. While single arm studies (with no control) can be compelling if there is a strong enough signal for efficacy, a more statistically robust method is to generate synthetic control arms.
A synthetic control consists of a similar or matched patient set to the group receiving the experimental regimen, but these patients are not part of the trial. Instead, they are identified from various historical data sources such as real-world data, prior clinical trials, etc. and matched based on criteria such as biomarkers, clinical status, and other relevant stratifications.
The outcomes for these historical patients are compared to the outcomes for those receiving the experimental regimen. While this route eliminates the need for a control arm, it is perhaps less robust because one set of data is retrospective whereas the other is prospective.
However, the FDA has already begun accepting drugs conditionally based on efficacy from single arm studies based on superiority over the historical standard of care. For example, Roche’s Alecensa and Amgen’s Blincyto, both received accelerated approval based on single arm studies with synthetic control arms. Importantly, the FDA still typically requires a formal Phase 3 study to confirm an accelerated approval, but these approaches still provide significant time savings and get effective drugs to market faster.
Another approach for maximizing the control arms is to embed a cross-over design into the study. This means that at a certain point, typically once a participant on the control arm progresses, they switch over to the experimental arm and receive that regimen. This design has the benefit of assuring participants that they will have a chance at the experimental drug regardless of which arm they are assigned. While it can complicate data analysis because the experimental group will not necessarily have a superior survival outcome to the “control” group (because the control group also ultimately receives the experimental regimen as well), it can also be paired with a synthetic arm to bolster the analysis.
Signal-Maximizing, Adaptive Designs
In order to diversify beyond the classic 1 indication + 1 regimen model and to more rapidly confirm hypotheses about which pairing will be most beneficial, adaptive trials have expanded along two dimensions:
- Umbrella Design: Within 1 indication, multiple different regimens are attempted. As data is gathered on response vs progression for each regimen, participants who progress are shifted onto the regimens with emerging evidence for efficacy. This amplifies the potential signal and benefits participants who are getting a chance at a more beneficial option.
- Basket or Bucket Design: Here, a single regimen is attempted across multiple indications with some commonality in underlying biology or even a specific molecular alteration. While cross-over is not possible with this design, it is still quite beneficial for an organization to quickly learn which indications offer the best chances of success with a registrational trial. Pembrolizumab was approved for all MSI-H tumors and larotrectinib as well as entrectinib for all NTRK fusion containing tumors using this approach.
Value Demonstration
In an increasingly competitive market, organizations must convince insurers, pathway and guideline decision-makers, physicians, and patients of the value of their products, especially given the sometimes quite high prices. Proof of value to payers is particularly complex for diagnostic tests, which must prove that they impact treatment decision making and ultimately outcomes in a beneficial manner. For broad genomic tests, which rarely identify actionable findings beyond what would be found via indication-specific companion diagnostics (for example BRAF testing in melanoma), value demonstration can be even more challenging.
However, given the increasing interest in personalized medicine, both physicians and patients are demanding better access to panel-based tests. Diagnostics have a few possible approaches to propose to payers. One is to bundle the diagnostic with one or more drugs that can be recommended using the test. Another approach involves outcomes-based models where the test is reimbursed based on whether it delivers clinically-actionable results.
Similarly, some pharmaceutical companies are exploring outcomes-based pricing for their high-priced drugs. Although the deal ultimately did not work out, Novartis and Medicare/Medicaid recently explored a “pay-for-performance” model for the CAR-T therapy Kymriah, which costs nearly $500k but provides an essentially curative outcome for many patients with leukemia. We expect further proof of concept models to launch soon.
Conclusion
Over the next 5 years, we anticipate transformative improvement in outcomes for people with cancer, driven not just by better products but by improved access as well. Both diagnostic and pharmaceutical companies will drive this change, based on foundations of scientific rigor, development creativity and flexibility, and robust organizational capabilities.